Precision agriculture practices involve more precise seeding, irrigation, fertilization, and pesticide use in order to optimize crop production for the purpose of increasing grower revenue and reducing the impact of agriculture on the environment simultaneously. In this article, we will discuss the use of PA practices, such as variable rate irrigation and remote sensing, in potato production.
According to the International Society of Precision Agriculture, “precision agriculture (PA) is a management strategy that gathers, processes and analyzes temporal, spatial and individual data and combines it with other information to support management decisions according to estimated variability for improved resource use efficiency, productivity, quality, profitability and sustainability of agricultural production.”
In other words, PA provides the possibility to do the right thing, in the right place, at the right time and in the right way. High-value crops like potatoes are recognized as good candidates for the adoption of PA because of the high cost of inputs. In addition, the sensitivity of potato tuber yield and quality to production practices and environmental conditions makes precision management economically critical.
Variable rate irrigation
The variable rate irrigation (VRI) technology applies water at variable rates rather than one uniform rate along the length of the center pivot. There are two steps to apply the VRI: firstly, based on soil electrical conductivity (EC) or elevation mapping, the field is divided into different management zones; secondly, the system applies specific amount of water on different management zones by turning on and off individual nozzles (nozzle control VRI) or controlling the moving speed of the pivot (speed control VRI).
VRI can apply water at differing rates to different crops or cultivars, varying soil types, high run-off areas or low areas prone to getting wet and saturated, and environmentally sensitive areas within the field. The overarching goal of VRI is to avoid over- and under-irrigation so no water is wasted and no water stress occurs, while crop yield and quality are maintained or increased.
In the summer of 2018, we conducted a study to quantify the benefits of using VRI on commercial potato (Russet Burbank) production in Wisconsin. We picked two fields irrigated with nozzle control VRI and speed control VRI respectively.
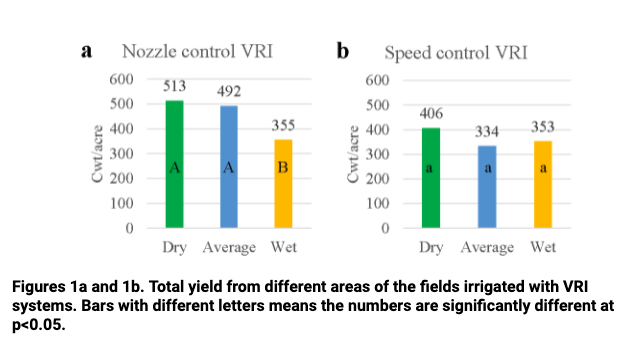
In each field, there was about 15 feet elevation difference between the highest and lowest areas. At harvest, we evaluated tuber yield and quality of the driest area, the most representative/average area, and the wettest area of each field. Under nozzle control VRI (Figure 1a), there was a significant yield reduction (about 140 cwt/a, p<0.05) in the wettest area compared to the average area. Yield from the driest area was slightly higher (about 20 cwt/a, p>0.05) than that from the average area. Under speed control VRI (Figure 1b), there was not any significant yield difference between the three locations, although the number in the driest area was higher than those in the average and wettest areas.
Our data suggested that:
- A big benefit of using VRI is to improve yield and quality, therefore to potentially improve the profitability in the direst (or the high run-off) area of a field that is more vulnerable to under-irrigation. VRI is able to maintain soil moisture within the rooting zone of the plants;
- VRI can save irrigation water and improve irrigation efficiency in the low area of a field that tends to be wet or saturated. However even under VRI, managing potato yield and quality in the low area is still challenging, since plants tend to have more rotting and defect issues;
- VRI is a promising system to save water while improving potato profitability, but further fine-tuning is needed to better manage it on fields with variability.
Remote sensing and machine learning
To maintain yield and profitability, potato farmers must satisfy the need of the crops for nitrogen. To minimize environmental degradation and decrease the financial risks associated with regulatory and legal uncertainty surrounding nitrate in groundwater, potato farmers need new management tools to help them apply the right amount of nitrogen at the right time throughout the growing season.
Commonly used methods of monitoring in-season potato plant nitrogen status are labor-intensive, time-consuming, sometimes misleading, and are only site-specific within the field. In addition, there are no publicly available tools that generate whole field maps to predict in-season plant nitrogen status and end-of-season tuber yield using remote sensing imagery.
Remote sensing is an innovative, timely, non-destructive and spatially comprehensive approach to improve existing in-season crop production management practices. Remote sensing typically provides several narrow spectral bands (~ 3-10 nm), which can capture fine absorption features of crop nutrients. So far many studies have indicated that remote sensing can be effectively applied to predicting crop parameters/variables, such as leaf area index, biomass, foliar N concentration, etc.
The methods used to predict/model crop traits mainly focus on building predicting algorithms between spectral signals and field measurements. A typical model predictor is vegetation indices (VI), which are mathematical combinations of reflectance at two or more spectral bands. For example, normalized difference vegetation index (NDVI) has been widely used in previous studies due to its simple application to monitoring vegetation dynamic at regional and global scales.
We have studied on three machine learning models (decision tree (DT), support vector machine (SVM) and random forest (RF)) that used NDVI to predict the N status and final yield of four potato cultivars (two russets including Silverton and Lakeview Russet, two chippers including Snowden and Hodag) over two growing seasons in 2018 and 2019.
Our preliminary results indicated that NDVI has great potential of predicting the potato N status indicated by petiole NO3-N, whole leaf total N, or whole vine total N as well as the end-of-season total yield (Table 4). We used R2 that ranges from 0 to 1 to measure goodness-of-fit for the models. The higher the R2, the better the prediction. It is considered to be a very good prediction if R2 is higher than 0.75.
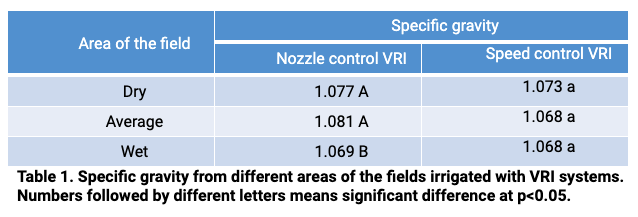
Under both VRI systems, tubers from the wettest area had lower specific gravity compared to those from the driest and average areas, and the difference under the nozzle control system was significant (Table 1).
Figure 2 showed that tubers from the wettest area of each field had significantly higher length to width ratio. Correspondingly, there was higher incidence of
hollow heart in tubers from the wettest areas of both fields, and the difference was significant under the speed control system (Table 2).
During storage under 48°F, we observed higher incidence of rotting in tubers from the wettest areas of both fields (Figure 3). It is thought that the tubers grown in saturated soils had enlarged lenticels on the surface, which created perfect entry points for pathogens in field and during storage.
In addition, we calculated irrigation efficiency (IE) of the speed control VRI system (numbers of the nozzle control VRI were not available), and it showed that there was a significant improvement of IE in the wettest area compared to the average area of the field. IE in the driest area was slightly higher than the average (Table 3).
For nitrogen status, using NDVI to predict petiole nitrate-N generated the best R2 results for both potato types, compared to whole leaf total N and whole vine total N. For total yield prediction, DT and RF were better than SVM, and the results for 2019 were better than 2018 (Table 4, above).
So far we have found that:
- 1) decision tree and random forest are better than support vector machine for predicting both in-season N status and end-of-season yield for potatoes;
- 2) petiole nitrate-N can be better predicted using NDVI and machine learning models compared to total N in leaves or vines. We will validate the models and expand this work using more years of data on more potato varieties.
The author would like to thank the Wisconsin Potato and Vegetable Growers Association, the Wisconsin Department of Agriculture, Trade and Consumer Protection, the Wisconsin Fertilizer Research Council, and the University of Wisconsin-Madison College of Agricultural and Life Sciences for funding our research.
— Yi Wang is an assistant professor in the University of Wisconsin-Madison’s Department of Horticulture. She is a former winner of Spudman’s Emerging Leader Award.