Drones can help potato growers quickly detect alternaria. This was stated by Ruben van de Vijver of the Institute for Agricultural, Fisheries and Food Research (ILVO) during the Belgian potato fair Interpom.

ILVO has been experimenting for some time with detecting alternaria with sensors, drones and artificial intelligence. “We try to give the grower more information, a better overview, via the drone,” says the researcher. It won’t come to the point where artificial intelligence can replace the grower’s expertise altogether, he added.
Early stage
Fungal stains can also be quickly detected via drones. “Sensors on the drones help to detect the disease in the field as early as possible,” says Van de Vijver. “Thanks to artificial intelligence, you can already see the fungal disease on one plant. That is much faster than the grower can see, he usually only sees it when large areas are infected. It is important to kill the disease once it is in your plant and the earlier you catch it, the more you can limit its impact. Thanks to this early detection, the grower can intervene effectively with targeted spraying and with limited environmental and financial impact.”
Results via tablet
Thanks to this technology, the arable farmer receives more information and an overview of where the disease is in the field via a tablet. The intention is that he can spray less and more precisely. “The grower can skip spraying or postpone it for a few days. Potatoes are sprayed between 10 and 18 times, depending on the weather. If you can postpone a spraying for two days, that means a huge profit.”
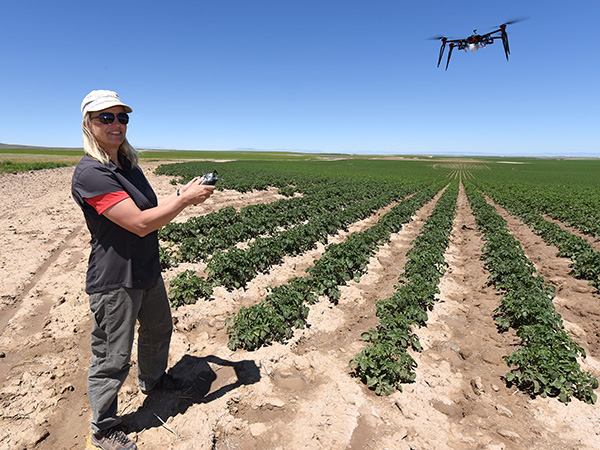
Automatic detection of early blight caused by Alternaria solani could promote a drastic reduction in the consumption of plant protection agents and the related production losses. A proximal sensing platform was constructed and calibrated for acquiring high resolution hyperspectral images in the field, and used to accurately map Alternaria lesions. High resolution canopy reflectance images were obtained for 32 potato plants that had been infected with A. solani and 32 healthy reference plants.
Spectral classifiers like partial least squares discriminant analysis (PLS-DA) and support vector machines (SVM) based on PCA scores were tested to discriminate affected and non-affected pixels. Both spectral classifiers performed well at pixel level with accuracies above 0.92. The NIR region (750 nm) was identified as the most discriminative part of the spectrum for detecting the lesions. As the disease pressure is typically expressed as the number of lesions per area, the accuracy was also evaluated at this level.
This indicated a considerable number of false detections at the edges of the leaves and the leaf axils. Therefore, a decision tree was designed based on expert knowledge about the shape of Alternaria lesions, and used to post-process the classified images. This reduced the number of false detections, increasing the precision from 0.17 to 0.22 at the expense of a reduction in recall from 0.88 to 0.84. This leaves considerable room for improvement in the classification accuracy at the object level. We learned that (1) few, broad wavelengths are sufficient and (2) spatial context is essential for the detection of lesions caused by Alternaria infection.
The application of more powerful object classification techniques such as convolutional neural networks to enhance the model performance by efficiently encapsulating the spatial context in the classifier might further improve the detection performance. This could pave the way to UAV or tractor based Alternaria mapping.